Big Data Improves Renewable Power Forecasts. Will It Help India?
by and -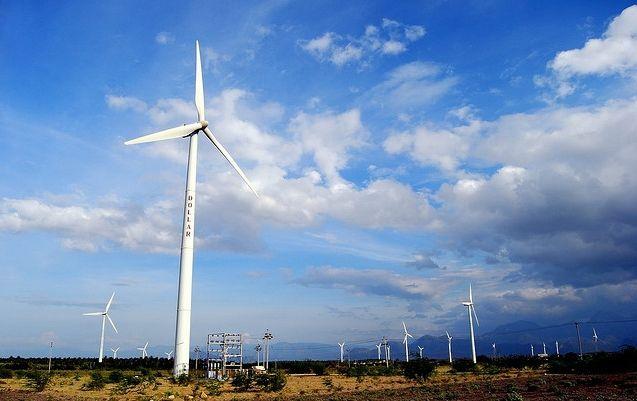
This blog originally appeared on Insights
India is one of the world’s fastest-growing markets for renewable energy. The government has set ambitious renewable energy targets for this year of 175 gigawatts by 2022, an increase of 400 percent over 2014, with 100 GW from solar photovoltaics and another 60 GW from wind. But even as India looks to add these new wind and solar plants, it is working to absorb the renewable power it already generates.
India’s leading wind power state, Tamil Nadu, is a case in point. Despite significant available wind energy, the limited ability to anticipate how much wind power will be generated in the next 24 hours has meant that conventional power plants keep running and clog transmission lines. These plants could be ramped down or even shut down, with more wind power sent to customers, if grid operators had better forecasts.
Why does forecasting matter? The answer begins with the basic job of grid operators, which is to balance electricity supply and demand, every minute of the day. To do this, grid operators schedule power generation one day in advance, committing the power plants under their authority to an hour-by-hour timetable of when to run and supply electricity. Getting that balance wrong results in blackouts or power surges.
This daily balancing commitment is partly for technical reasons -- some types of power plants, notably coal, take more time to warm up – but it’s also partly economic, because fossil generation companies don’t want to generate power and burn expensive fuel without a guarantee of being paid. The other important factor is the availability and capacity of the transmission network, to transmit power from generation plants and send it to consumers. Network traffic jams can make this difficult.
Not Just Variable – Unpredictably Variable
All this works great, if both supply and demand are perfectly predictable. Decades ago, the unpredictability was mostly on the demand side: grid operators knew how much coal, large hydro, nuclear and gas power they could turn on, but they didn’t know for sure how much power their customers would use the next day.
Over time, increasingly accurate computer models based on historical data and other factors were able to predict how much power consumers would use. Today’s load forecasting models in several parts of the world have an impressively low error rate of less than 5 percent.
But just as the demand-side predictions were getting under control, there was emerging complexity in the supply side, in the form of variable renewable energy technologies like wind and solar. In addition to their environmental benefits, significant technology advances and cost reductions have made renewables the prime energy choice for several countries.
The problem is that these technologies aren’t just variable, they are unpredictably variable. It’s relatively hard to know how much energy those power plants will generate the next day. As a result, grid operators need to identify and commit additional power plants – usually fossil-based – as back-ups. In small quantities, this is identical to the extra back-up resource needed for fossil fuel plants. But the more uncertainty there is about wind and solar, the more back-up plants are needed, and the larger the cost.
This is where the real value of renewable energy forecasting comes in. Researchers have been working hard for years on computer models that can predict more accurately how much wind and solar power will be generated the next day, hour by hour. These models use weather data, historical patterns, information about the technical features of the wind and solar plants, and other data to calculate predictions of generated power.
As these models get more accurate, grid operators can reduce the amount of back-up generation they need to keep online, and thus reduce costs. A 10 percent improvement in accuracy of wind forecasting on the western U.S. grid would save tens of millions of dollars per year, increasing to $100 million dollars per year for higher levels of wind penetration. Colorado-based Xcel Energy, an early adopter of wind forecasting models, saw $40 million in savings over four years.
Pitfalls and Promise for Renewables Forecasting in India
Unfortunately, India’s adoption of advanced wind and solar forecasting has been slow. In 2013, the Central Electricity Regulatory Commission (CERC) tried to address this problem by requiring all wind and solar plant operators to make day-ahead predictions for the amount of power they would generate. Wind plant predictions that were off by more than 30 percent triggered a fine.
Wind power developers objected, arguing that the technology wasn’t ready yet, so it wasn’t possible to comply, and the rule was suspended in 2014. This year, CERC introduced a more robust draft framework to strengthen forecasting in the renewables sector.
During this period, India’s National Institute of Wind Energy has been working to introduce more accurate wind forecasting. Recently, it has partnered with Barcelona-based wind modeling company Vortex to support Tamil Nadu’s state-owned utility Tangedco to deliver accurate wind forecasting. This effort appears to be making progress, although the system has yet to be completely implemented.
So now the question is whether this promising pilot effort at wind forecasting can be scaled up to cover most or all of India’s wind power generation, and also if advanced forecasting models can achieve the same levels of accuracy in India as in other countries. If so, it will help put the country on a firmer footing to achieving its ambitious renewable energy targets.